Project Title
Development of a hybrid neutral network forcthe classification of embryonic growth stages and malformations
Research Team
Contact Information
Phys. Abner Chica Herrera
External colaborator: Dr. Hernán Morales Navarrete
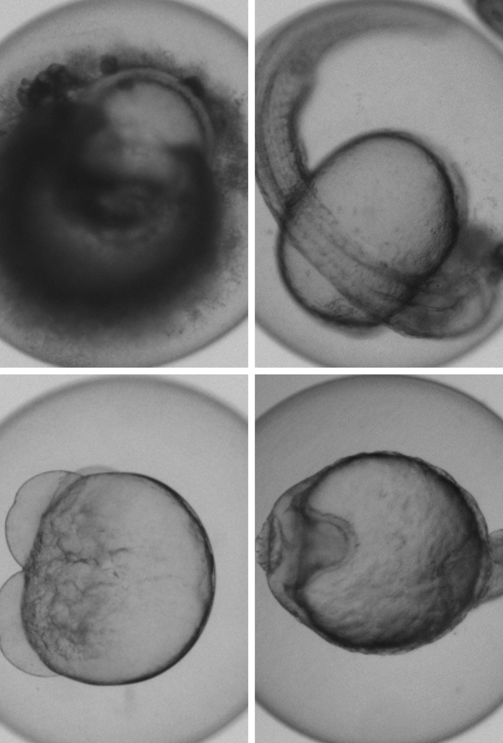
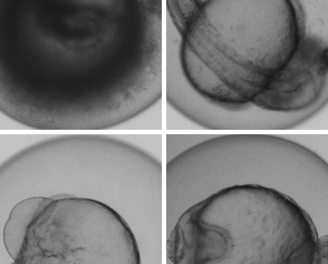
Institutional e-mail:
Overview
Predicting morphological events during early embryonic development presents significant challenges in developmental biology research. This study introduces an innovative computational methodology that integrates hybrid neural network architectures, combining pre-trained convolutional neural networks (ResNet-50, ResNet-18) with quantum computational layers for embryonic image analysis. Using zebrafish (Danio rerio) as a biological model, we developed a sophisticated machine learning approach specifically designed to address small dataset limitations through quantum-classical integration. The research presents three key contributions: a novel hybrid quantum-classical neural network architecture, advanced image processing algorithms, and a robust multiclass morphological classification methodology. This computational framework enhances predictive performance in embryonic malformation detection, offering a generalizable approach with broad applications across biological research domains. The integration of quantum computing principles with traditional machine learning techniques establishes a robust foundation for early-stage developmental anomaly detection and predictio.
Alternative e-mail:
abner.chica@czetesis.com
abner.242000@hotmail.com